DSAG: A Scalable Deep Framework for Action-Conditioned Multi-Actor Full Body Motion Synthesis
Abstract
- We introduce DSAG, a controllable deep neural frame-work for action-conditioned generation of full body multi-actor variable duration actions.
- To compensate for incompletely detailed finger joints in existing large-scale datasets, we introduce full body dataset variants with detailed finger joints.
- To overcome shortcomings in existing generative approaches, we introduce dedicated representations for encoding finger joints.
- We also introduce novel spatiotemporal transformation blocks with multi-head self attention and specialized temporal processing.
- The design choices enable generations for a large range in body joint counts (24 - 52), frame rates (13 - 50), global body movement (inplace, locomotion) and action categories (12 - 120), across multiple datasets (NTU-120, HumanAct12, UESTC, Human3.6M).
- Our experimental results demonstrate DSAG’s significant improvements over state-of-the-art, its suitability for action-conditioned generation at scale.
Architecture
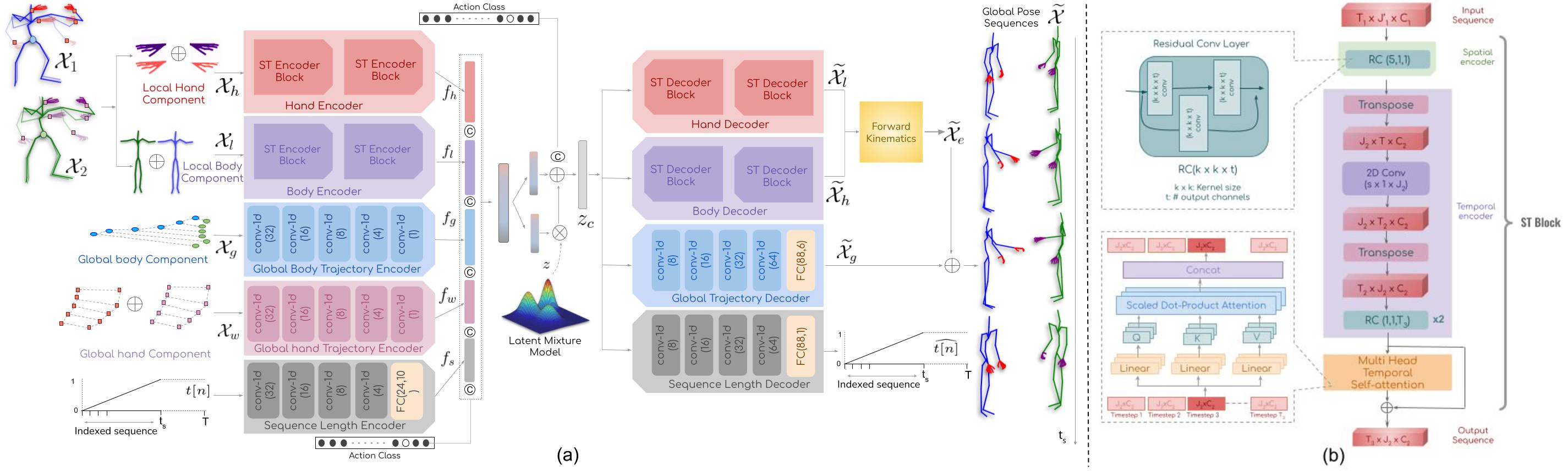
Quantitative Comparision
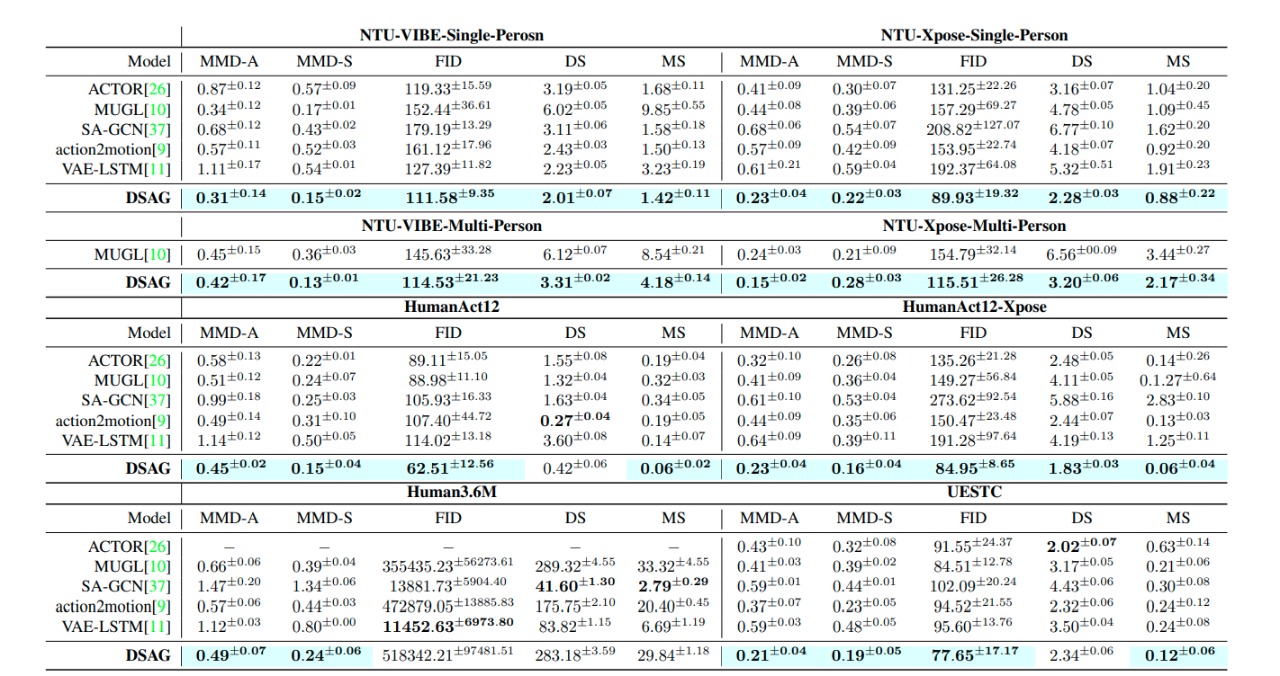
Qualitative Comparision
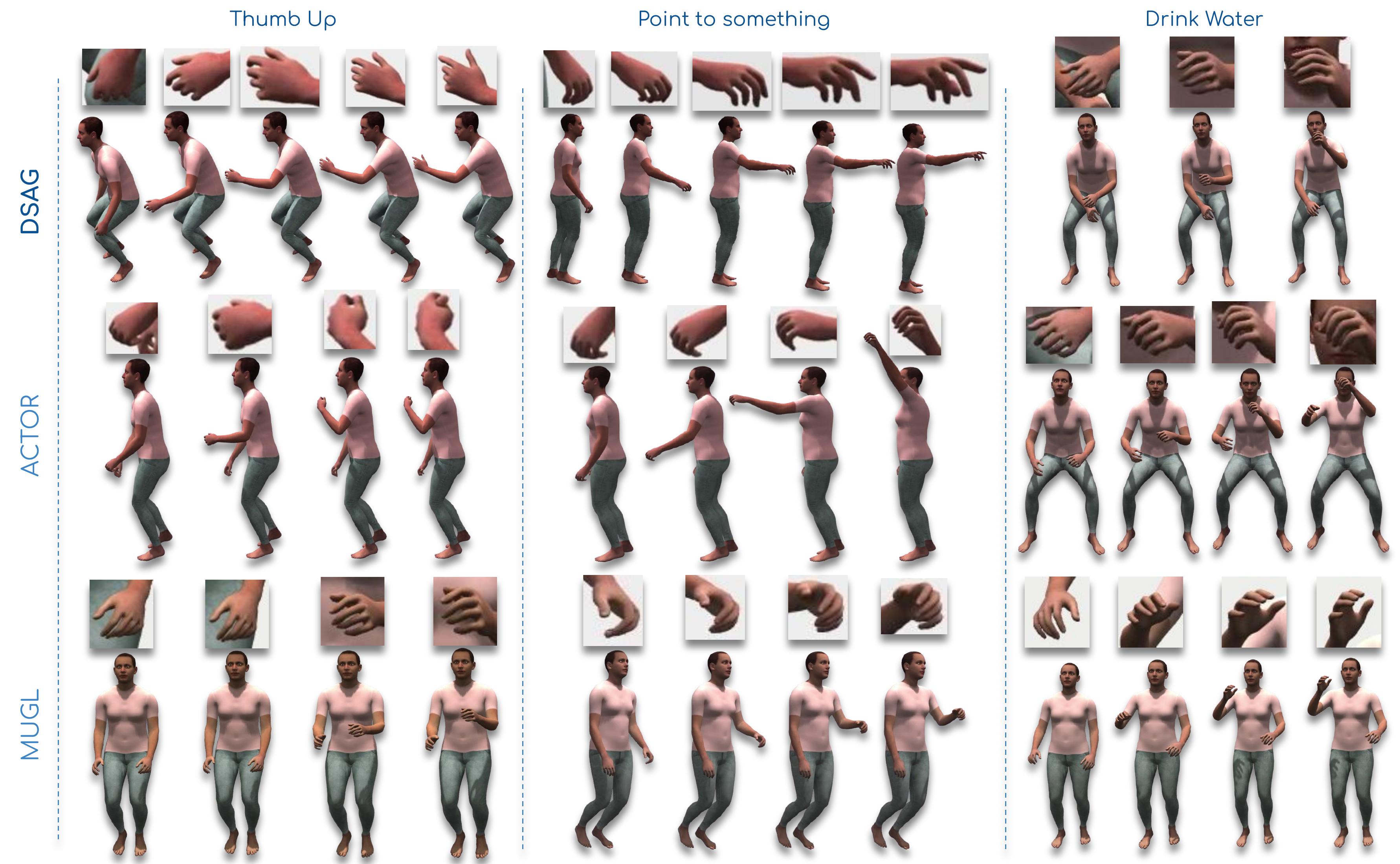
Citation
Please cite our paper if you end up using it for your own research.
@InProceedings{DSAG, author = {Gupta, Debtanu and Maheshwari, Shubh and Kalakonda, Sai Shashank and Manasvi and Sarvadevabhatla, Ravi Kiran}, title = {DSAG: A Scalable Deep Framework for Action-Conditioned Multi-Actor Full Body Motion Synthesis}, booktitle = {Proceedings of the IEEE/CVF Winter Conference on Applications of Computer Vision (WACV)}, month = {January}, year = {2023} }