NTU-X: An Enhanced Large-scale Dataset for Improving Pose-based Recognition of Subtle Human Actions
The lack of fine-grained joints (facial joints, hand fingers) is a fundamental performance bottleneck for state of the art skeleton action recognition models. Despite this bottleneck, community's efforts seem to be invested only in coming up with novel architectures. To specifically address this bottleneck, we introduce two new pose based human action datasets - NTU60-X and NTU120-X. Our datasets extend the largest existing action recognition dataset, NTU-RGBD.
In addition to the 25 body joints for each skeleton as in NTU-RGBD, NTU60-X and NTU120-X dataset includes finger and facial joints, enabling a richer skeleton representation. We appropriately modify the state of the art approaches to enable training using the introduced datasets. Our results demonstrate the effectiveness of these NTU-X datasets in overcoming the aforementioned bottleneck and improve state of the art performance, overall and on previously worst performing action categories.
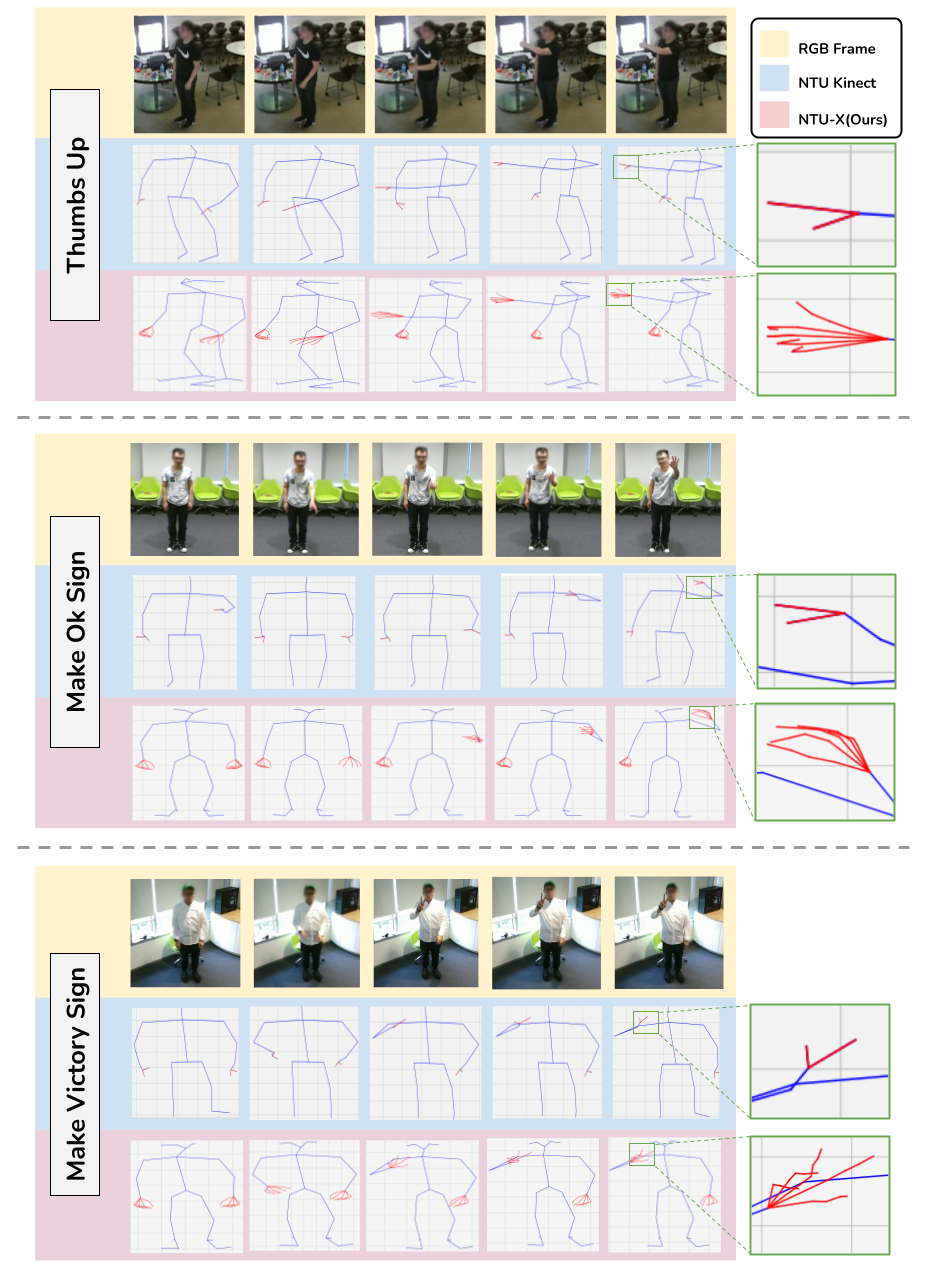
Please cite our paper if you end up using it for your own research.
@misc{trivedi2021ntux, title={NTU-X: An Enhanced Large-scale Dataset for Improving Pose-based Recognition of Subtle Human Actions}, author={Neel Trivedi and Anirudh Thatipelli and Ravi Kiran Sarvadevabhatla}, year={2021}, eprint={2101.11529}, archivePrefix={arXiv}, primaryClass={cs.CV} }