Syntactically Guided Generative Embeddings for Zero-Shot Skeleton Action Recognition
We introduce SynSE, a novel syntactically guided generative approach for Zero-Shot Learning (ZSL). Our end-to-end approach learns progressively refined generative embedding spaces constrained within and across the involved modalities (visual, language). The inter-modal constraints are defined between action sequence embedding and embeddings of Parts of Speech (PoS) tagged words in the corresponding action description. We deploy SynSE for the task of skeleton-based action sequence recognition. It has been accepted for publication at the 2021 IEEE ICIP.
Our design choices enable SynSE to generalize compositionally, i.e., recognize sequences whose action descriptions contain words not encountered during training. We also extend our approach to the more challenging Generalized Zero-Shot Learning (GZSL) problem via a confidence-based gating mechanism. We are the first to present zero-shot skeleton action recognition results on the largescale NTU-60 and NTU-120 skeleton action datasets with multiple splits. Our results demonstrate SynSE’s state of the art performance in both ZSL and GZSL settings compared to strong baselines on the NTU-60 and NTU-120 datasets.
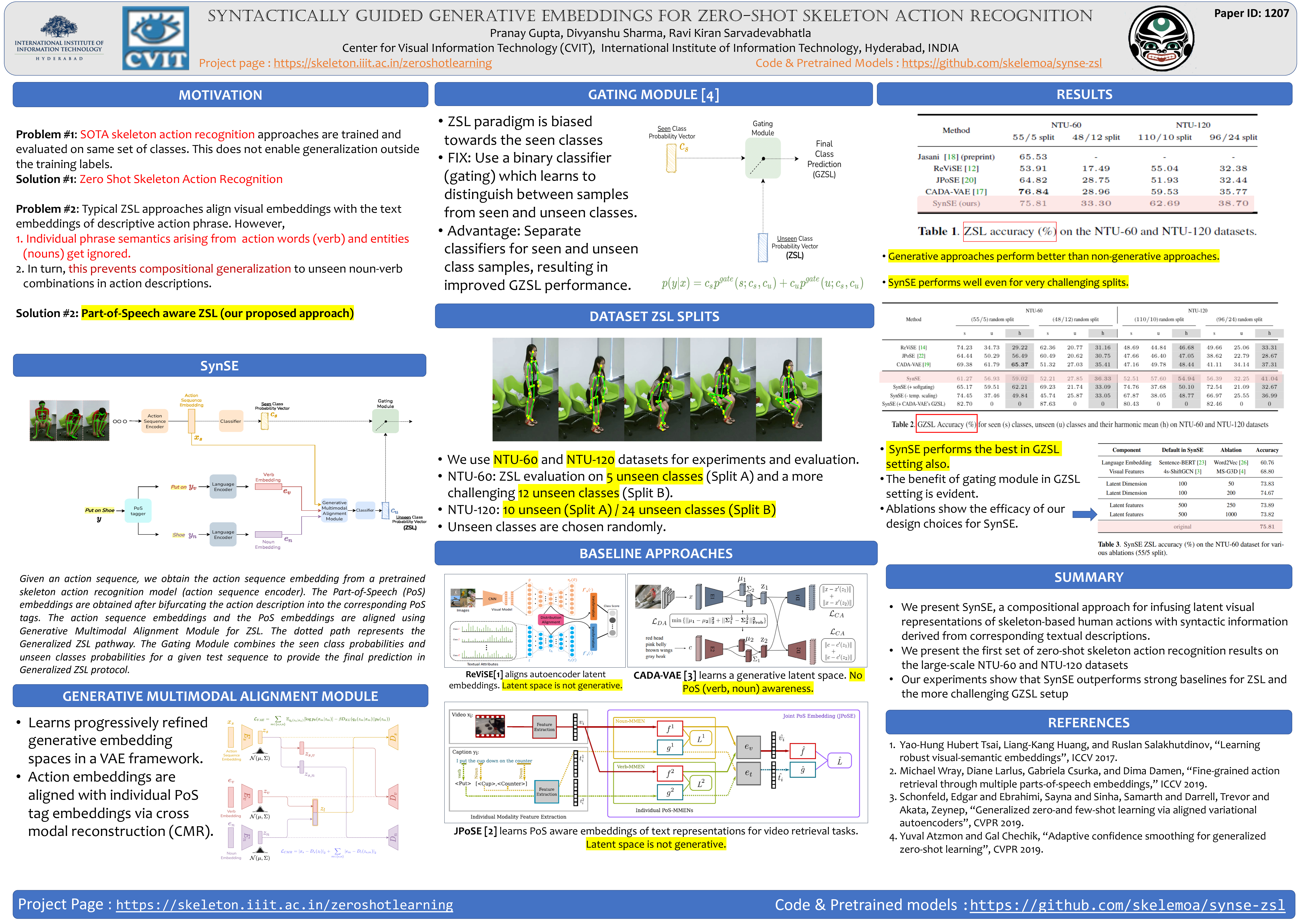
Please cite our paper if you end up using it for your own research.
@misc{gupta2021syntactically, title={Syntactically Guided Generative Embeddings for Zero-Shot Skeleton Action Recognition}, author={Pranay Gupta and Divyanshu Sharma and Ravi Kiran Sarvadevabhatla}, year={2021}, eprint={2101.11530}, archivePrefix={arXiv}, primaryClass={cs.CV} }