
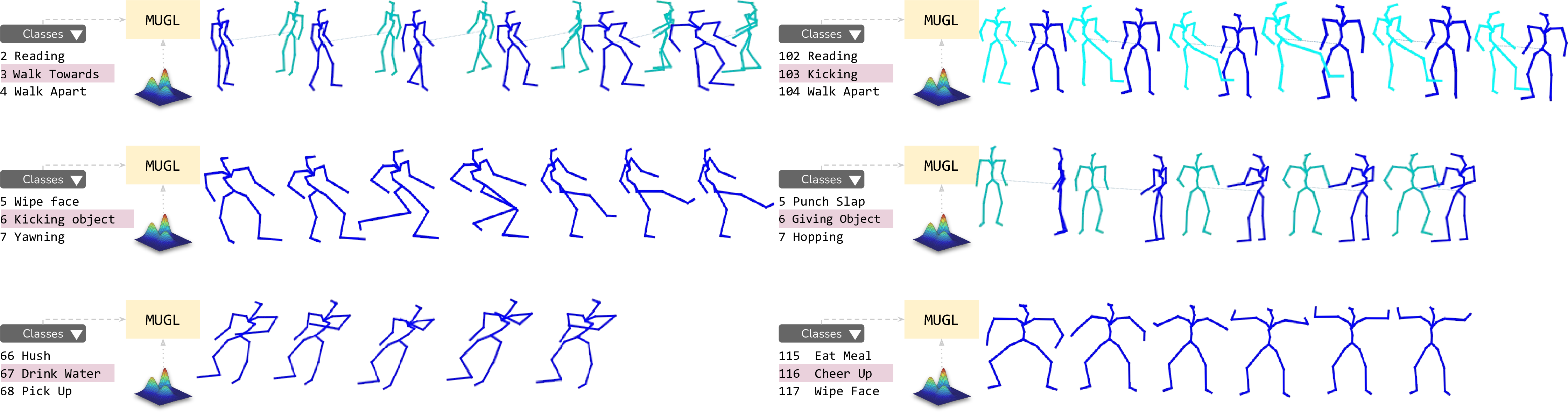
Abstract
- We introduce MUGL, a novel deep neural model for large-scale, diverse generation of single and multi-person pose-based action sequences with locomotion.
- Our controllable approach enables variable-length generations customizable by action category, across more than 100 categories.
- To enable intra/inter-category diversity, we model the latent generative space as a Conditional Gaussian Mixture Variational Autoencoder.
- To enable realistic generation of actions involving locomotion, we decouple local pose and global trajectory components of the action sequence. We incorporate duration-aware feature representations to enable variable-length sequence generation.
- We use a hybrid pose sequence representation with 3D pose sequences sourced from videos and 3D Kinect-based sequences of NTU-RGBD-120.
- To enable principled comparison of generation quality, we employ suitably modified strong baselines during evaluation. Although smaller and simpler compared to baselines, MUGL outperforms the baselines across multiple generative model metrics.
- Code will be made available.
Architecture
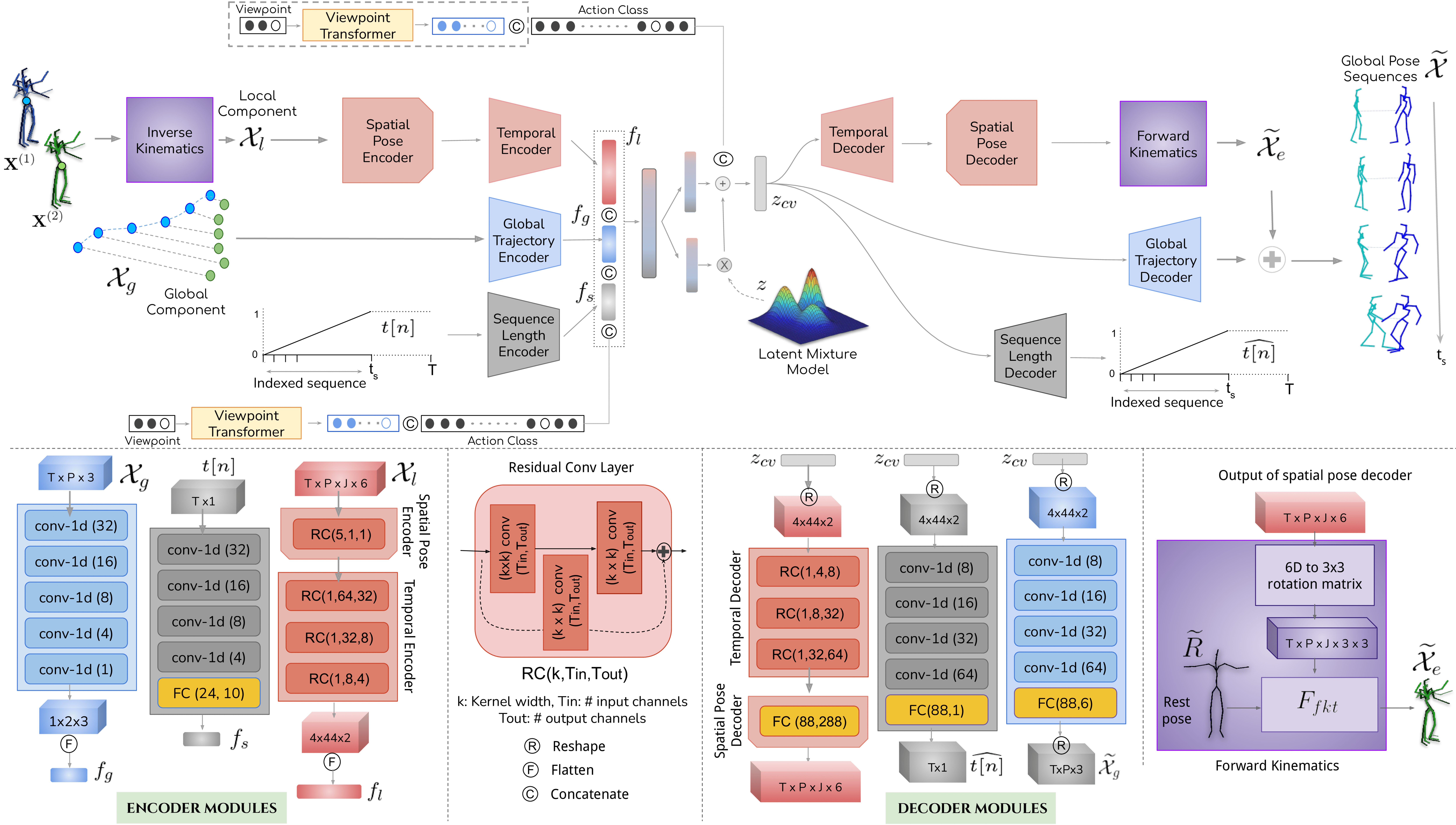
Generated Samples
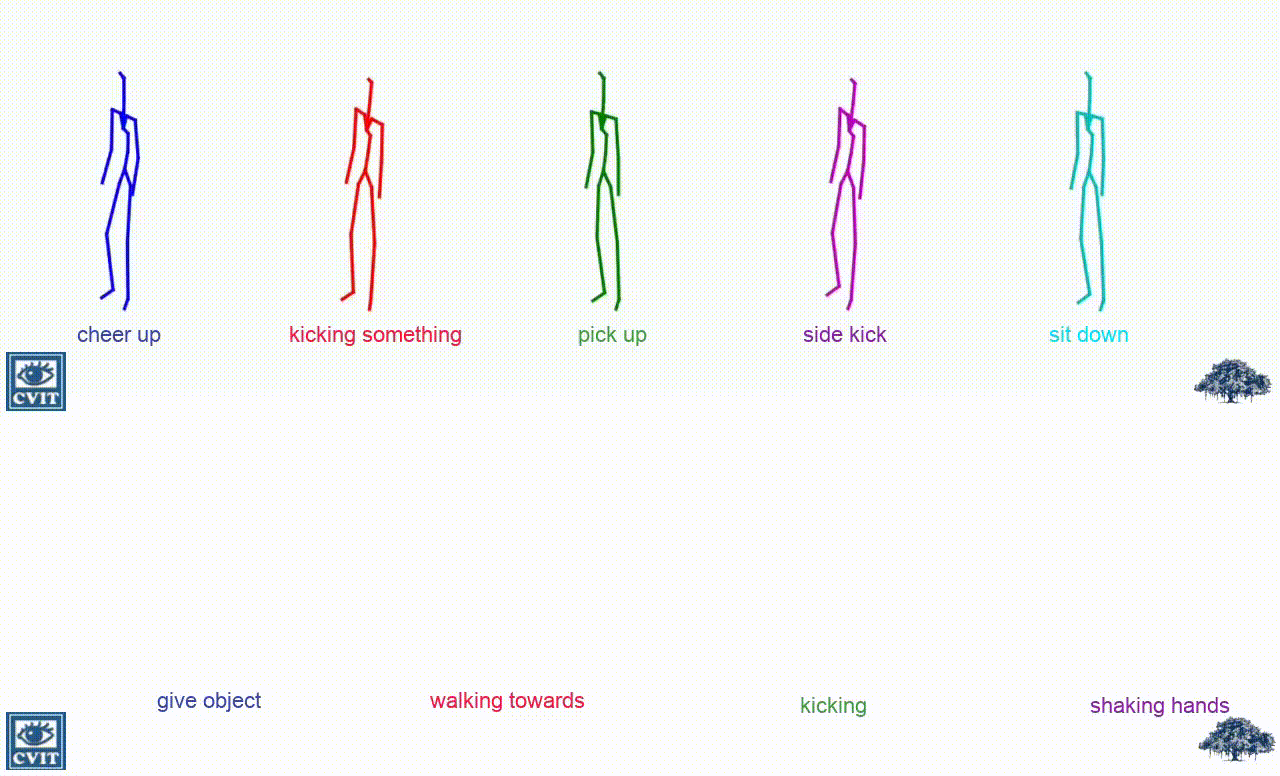
Quantitative Comparision

Qualitative Comparision
Issues with feature based generative quality measures
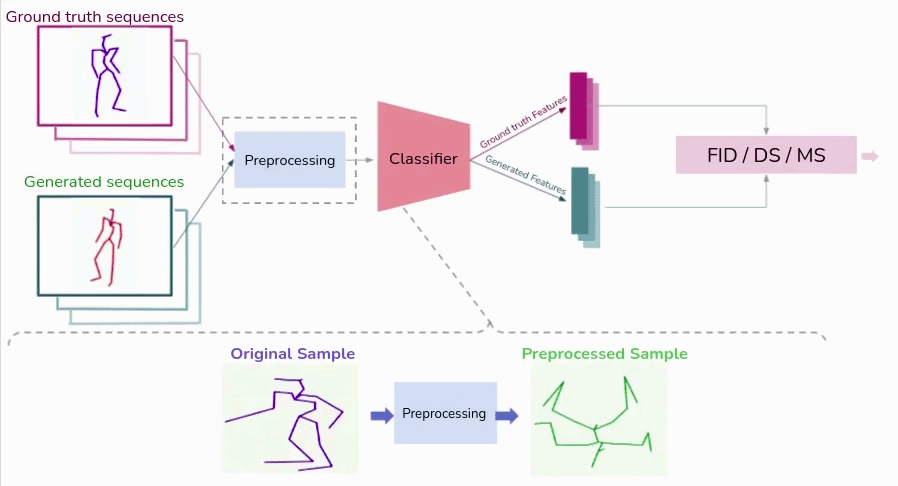
Citation
Please cite our paper if you end up using it for your own research.
@InProceedings{Maheshwari_2022_WACV, author = {Maheshwari, Shubh and Gupta, Debtanu and Sarvadevabhatla, Ravi Kiran}, title = {MUGL: Large Scale Multi Person Conditional Action Generation With Locomotion}, booktitle = {Proceedings of the IEEE/CVF Winter Conference on Applications of Computer Vision (WACV)}, month = {January}, year = {2022}, pages = {257-265} }